Caplena & ChatGPT: A Comparison in Text Analysis and Topic Assignment

Picture this: In the vibrant atmosphere at our recent conference, a question danced on everyone’s lips:
Our answer to this is a mix of “yes” and “no.” At Caplena, we primarily use our custom AI model for core tasks and ChatGPT for the summarization feature. Drawing a parallel between Caplena and ChatGPT in text analysis might seem like a riddle wrapped in a mystery. But it’s more like contrasting a powerful motor with a cutting-edge vehicle. Each comes with a unique prowess and purpose, aptly suiting distinct scenarios. But are they identical twins in the AI family? A resounding ‘no’ rings clear. Let’s delve into the reasons why👇.
ChatGPT, along with its GPT4 version, is a powerful tool, providing human-like responses to a broad array of questions. In contrast, Caplena, a feedback analysis platform, uses a specific AI model optimized for the task of categorizing large amounts of text data into distinct topics. Naturally, the question arises as to which tool offers a more effective analysis of customer feedback. In this study, we aim to compare the task of accurately categorizing sentences into relevant topics.
Task Definition:
Caplena vs. ChatGPT in Topic Assignment
To effectively compare Caplena and ChatGPT, we first need to define the task we’re evaluating. Our focus here is the process of topic assignment. Given a predefined set of topics, our goal is to determine their frequency of appearance in user feedback. To accomplish this, the AI needs to categorize each text into one or more relevant topics.
Fundamental Differences
Caplena: The Topic Maestro
The fundamental difference between Caplena and ChatGPT in topic assignment is that Caplena takes a direct approach, focusing specifically on topic assignment as its core task.
ChatGPT: The Chitchat Enthusiast
On the other hand, ChatGPT operates in a chat-like format, designed for engaging and interactive conversations. While ChatGPT can understand and respond to various questions, its primary objective is not that of topic assignment. Instead, ChatGPT aims to generate human-like responses, making it indirectly capable of addressing the task of topic assignment in the conversation.
ChatGPT’s User Interface
When comparing the user interfaces of ChatGPT and Caplena, ChatGPT utilizes a chat interface for receiving and generating conversational responses. Categorizing topics within sentences requires constructing a prompt in the form of a conversation, rather than directly inputting the text. Crafting a comprehensive prompt becomes crucial in ChatGPT’s approach. Let’s take a look at an example of how a prompt similar to this one might appear:
ChatGPT’s UI with Prompt vs. Caplena’s UI for Topic Assignment
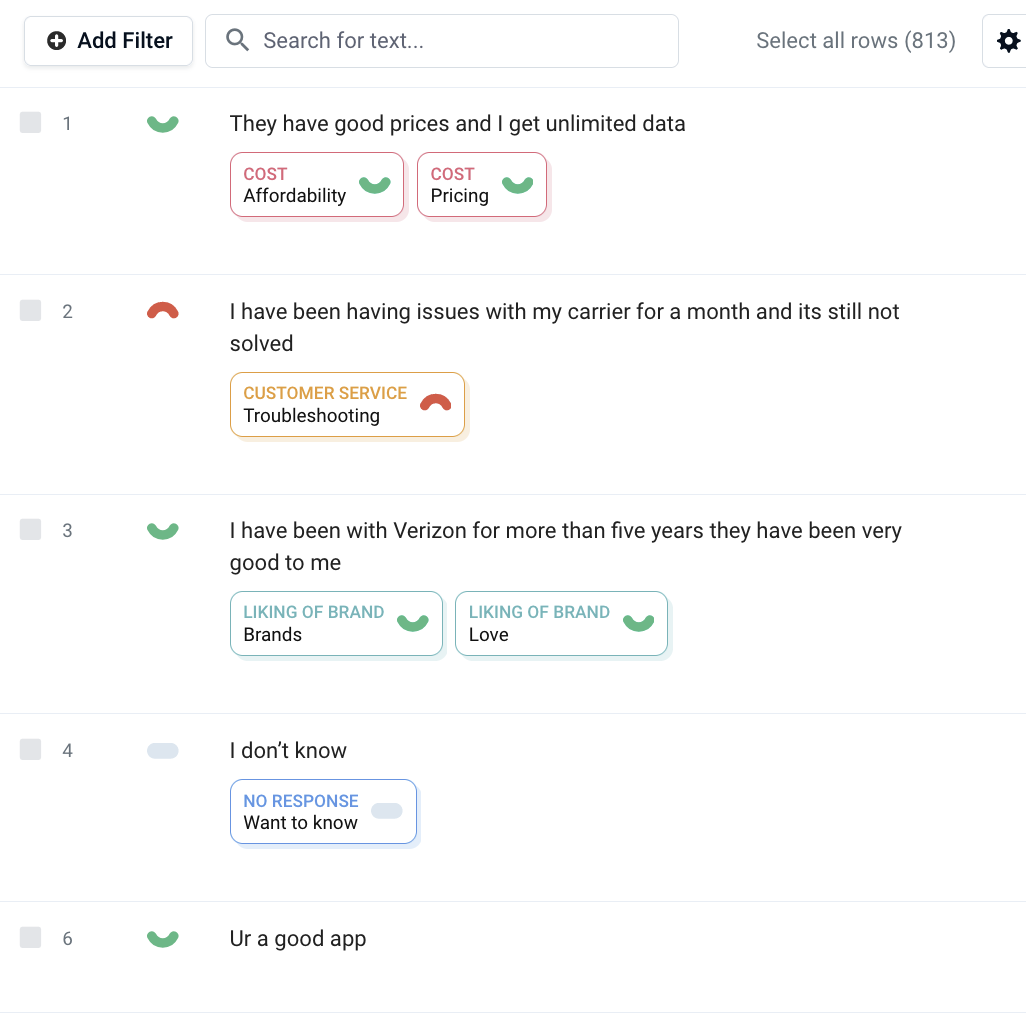
Topic Assignment Accuracy:
Caplena vs. ChatGPT
In our experiment, ChatGPT and Caplena went head-to-head, pitted against each other using a test dataset of 22 surveys. We employed the F1 score as our primary evaluation metric to assess the model’s accuracy on a scale of 0% to 100%. This metric measures how well the model aligns with a human-annotated dataset considering both precision and recall, which helps handle the uneven class distribution.
Our evaluation revealed that, overall, Caplena’s AI delivered superior accuracy. However, there were four instances where ChatGPT took the lead as illustrated below. Calculating the average F1 score across all 22 surveys, ChatGPT clocked in at 44%. Caplena’s AI scored a higher average of 56%. For reference, a naive model that randomly guesses will typically achieve an F1 score of approximately 5%. This difference is noteworthy despite a high degree of variance within the dataset. Although the numbers may seem similar at first glance, Caplena takes an additional step that significantly increases the percentage from 56%.
Caplena vs ChatGPT Performance
Fine-Tuning: The Caplena Advantage
Caplena’s standout feature lies in its remarkable fine-tuning capability. This enables a significant improvement from an initial F1 score of 56% to an ambitious target of 70% or higher. As mentioned earlier, a naive model that randomly guesses will typically achieve an F1 score of approximately 5%. A study by Ishita, Oard, and Fleischmann found that a fully manual human analysis only achieved an F1 score of 62.7%. This proves that humans are not infallible coders. Achieving a score of 70% places Caplena’s precision analysis on par with – or surpasses – a human-level performance. While the initial score of 56% shows good performance in many scenarios, it falls short of human accuracy. Especially in more complex scenarios like lengthy reviews or topics with semantic overlaps.
To overcome these challenges, we have implemented a streamlined process that actively involves users. In this process, users validate model outputs for a small subset of the dataset. Specifically, users review whether Caplena’s categorization is correct or incorrect for a select number of test cases. Working with the AI usually involves a couple of dozen rows. The manually validated data is then used to fine-tune the model to the specific task of the user, improving the model’s score on average from 56% to 66% in our setting, ensuring that it aligns with the user’s requirements.
To maintain transparency in our performance metrics, we use a portion of the human-validated data as a test set to estimate the F1 score. This evaluation method allows users to gauge the accuracy of the Caplena analysis. Users can monitor the evolution of their F1 score in real-time, providing insights into the model’s performance. We recommend considering an F1 score of 65 – 70 to indicate satisfactory accuracy. For a fair comparison, we used manually validated samples to also enhance ChatGPT. In particular, we employed a technique called few-shot learning. This involves including some examples with solutions in the prompt. However, ChatGPT struggled to benefit from this extra information in the prompts. This resulted in a decrease in ChatGPTs overall score from 44% to 41%.
Caplena AI After Fine-Tuning vs ChatGPT after fine-tuning
F1 Score Outcomes
Caplena made significant performance improvements through an interactive process in this specific study.
- Caplena’s F1 score increased from 56% to 66% with just a few human inputs per survey. To ensure a fair comparison, we applied a similar fine-tuning process to ChatGPT using few-shot learning.
- ChatGPT’s F1 score decreased from 44% to 41% despite using the same data for fine-tuning. This indicates that this method instead yields a negative performance for ChatGPT.
Caplenas Fine-Tuning Process
Study Limitations and Conclusion
Our study encountered challenges due to ChatGPT’s prompt size constraint. This limited the number of verified samples that could be included in the few-shots learning prompt and hindered a higher F1 score. Thus, we had to cap Caplena’s F1 score at 66%, while ChatGPT achieved 41%, ensuring fairness in the experiment. However, it should be noted that Caplena can achieve scores of 70 and above in real-life applications.
Even without few-shot learning, we observed that ChatGPT struggles with very long prompts. While the hard limit of 3000 words in ChatGPT was sufficient to analyze all the surveys in our sample, we noticed a decline in performance when analyzing questions covering a wide range of topics. In contrast, Caplena’s model, specifically trained for this task, does not suffer from this issue in surveys with many diverse topics.
Fortunately, the successor model, GPT4, is capable of handling much longer sequences, up to 20,000 words. This development has us excited to test it out. However, at present, the API for GPT4 is slow, and frequent lockouts occur due to the high volume of requests. We eagerly anticipate analyzing the model in the future.
Also, there were instances where ChatGPT struggled to interpret our prompts correctly. This resulted in responses like:
“Cannot classify the 35th review as it seems to be incomplete or unrelated to the topic list.”
“Little confused by this review, it doesn't seem to provide enough information to assign a topic. Can you provide more context or information to help me understand the review better?”
“1,2,3 all have [TOPIC]” (when multiple reviews are included in a prompt).
In sum: Caplena outperforms ChatGPT in the precisely defined text analysis tasks of topic assignment. Now, let’s unpack other elements contributing to Caplena’s unrivaled performance in the task of assigning topics to text.
A Quick Run-Through of Caplenas Workflow
Latest Feature: Summary Generation with ChatGPT
ChatGPT excels in text summarization, understanding and responding in a human-like manner to various prompts. Still, it is crucial to acknowledge its limitations in analyzing quantitative customer feedback topics. ChatGPT’s summarization feature is impressive, but it should not replace a comprehensive and precise textual analysis for optimal results.
- Recognizing the strengths of both tools, we have incorporated ChatGPT into Caplena’s latest feature: Summary Generation.
Unleash the power of data with our latest Summary Feature!
During this ongoing beta testing phase, we are effectively combining the best of both worlds. Caplena carries out the precise analysis, and ChatGPT provides a concise summary of the analyzed data. With this combination, one can quickly obtain a data overview in a few sentences, accessible with a single click. This ensures efficiency and a user-friendly experience, streamlining the data analysis process.
Caplenas New Summary Feature (in Beta Testing)
Conclusion
To wrap up, Caplena stands out as the superior choice for customer feedback analysis over ChatGPT for several key reasons:
- Zero-shot capability: Caplena surpasses Chat GPT by leveraging custom training data. Caplena’s zero-shot capability empowers models to generalize to new data or tasks based on their learned understanding of related information.
- Fine-tuning & quality assurance: Caplena’s fine-tuning feature dramatically improves its performance to an above-average human analysis. ChatGPT does not have this option.
- User friendly UI: Caplena offers a user-friendly interface specifically designed for textual analysis workflows, providing a smoother and more intuitive experience compared to ChatGPT’s chat-based interface. Additionally, while ChatGPT serves as a multipurpose algorithm, Caplena serves as a comprehensive solution tailored for a specific purpose.
- Privacy/Compliance: For many of our European customers, it’s currently not an option to send data to the US, making Caplena a safer choice from a data privacy perspective.
ChatGPT is an innovative AI tool that excels in generating human-like responses and offering contextual information. However, it is important to recognize both the strengths and limitations of ChatGPT. In the specific task of determining topics and their frequency of appearance in user feedback, Caplena clearly excelled. Caplena achieved an impressive overall score of 66%, surpassing ChatGPT’s 41% F1 score. Without the limitations imposed by this study, Caplena has the potential to achieve scores well beyond 70%, reaching above-average human-level accuracy. This is not surprising, given its purposeful design for the task, while ChatGPT serves a different use case.
Having said that, Caplena and ChatGPT are not in opposition; they complement each other. By integrating ChatGPT’s summarization feature into Caplena’s dashboard, users can leverage the strengths of both tools. This allows for valuable insights, utilizing powerful text analytics and visualization tools. This collaboration bridges the gap between sophisticated language models and advanced text analysis, expanding the boundaries of what can be achieved in this field.
So, is it really an “us vs them” question?
No. It’s an “us and them” opportunity for innovation and progress in the realm of text analytics.